Signalons l'étude que vient de publier McKinsey sur les données et leurs impacts stratégiques.
L'étude, très riche, est pleine d'enseignements sur les nouveaux "business models" à l'oeuvre dans les villes, notamment sur ceux de la mobilité. Par bien des aspects, elle rejoint nos analyses sur les "nouveaux modèles économiques urbains", dont les résultats seront publiés très prochainement, notamment sur le rôle des agrégateurs et la valeur permise par l'ajustement entre offre et demande en temps réel à grande échelle.
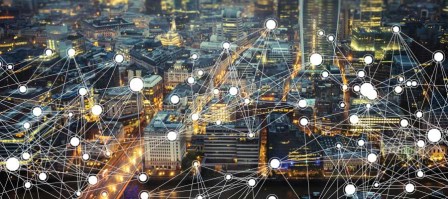
Extraits de l'étude à paraître sur les nouveaux modèles économiques urbains(Etude réalisée par ibicity, Acadie et Espelia ; financée par l'ADEME et l'AMF avec le soutien du PUCA).
L’activation de la multitude permet également d’ajuster en permanence l’offre et la demande de services urbains. Soit en jouant sur la demande, en compressant les pics de consommation de la ville via le mécanisme de l’effacement. Comme dans l’énergie – et plus largement comme dans l’ensemble des systèmes de flux -, la capacité à limiter les pics de circulation automobile via une meilleure information sur le trafic ou la capacité de report permet d’éviter la construction d’une nouvelle rocade sur un périphérique. Soit, par un mécanisme inverse, en jouant sur l’offre en mettant en vente la partie de l’infrastructure non utilisée – et donc en diminuant le dimensionnement de base et donc son coût. C’est l’exemple déjà cité de Zenpark qui permet d’utiliser les places de stationnement temporairement inutilisées, dans les parcs de stationnement publics comme privés, et permet ainsi de limiter le nombre de places de stationnement nécessaires. Là encore, le numérique n’est pas indispensable – le décalage du démarrage des cours fait par l’Université de Rennes est une forme d’effacement qui a permis de limiter la saturation de la nouvelle ligne de métro – mais il accentue ces possibilités d’ajustement entre offre et demande par cette capacité à produire des informations descendantes et remontantes, à la fois en temps réel et à grande échelle, et aussi par la capacité à fluidifier l’activation de la multitude (la technologie permet d’ouvrir les barrières des parkings).
L’activation de la multitude, la connaissance fine de l’usager et de l’usage, l’ajustement en temps réel de l’offre à la demande sont ainsi au cœur de l’optimisation du modèle économique des villes. Mais comment sont-elles rendues possibles ? La réponse tient en un mot : « plateformes » : celles-ci émergent comme une infrastructure additionnelle de la ville, à côté du modèle traditionnel des grands réseaux urbains.
Extraits de l'étude McKinsey (pages 57 et suivantes) (c'est nous qui soulignons - et nous avons gardé l'anglais !) :
HYPERSCALE PLATFORMS CAN MATCH SUPPLY AND DEMAND IN REAL TIME
Data and analytics are transforming the way markets connect sellers and buyers for many products and services. In some markets, each offering has critical variations, and the buyer prioritizes finding the right fit over the speed of the match. This is the case in real estate, for example, where buyers have strong preferences and finding exactly the right house is the priority. In others, the speed of the match is critical. “Hyperscale” digital platforms can use data and analytics to meet both types of needs.
These platforms have already set off major ripple effects in urban transportation, retail, and other areas. But that could be only the beginning. They could also transform energy markets by enabling smart grids to deliver distributed energy from many small producers. And they could make labor markets more efficient, altering the way employers and workers connect for both traditional jobs and independent work.
These platforms are already transforming the market for transportation
Hyperscale digital platforms can have notable impact in markets where demand and supply fluctuate frequently, where poor signaling mechanisms produce slow matches, or where supply-side assets are underutilized. These characteristics describe the status quo that prevailed in the taxi industry for many years before the arrival of Uber, Lyft, Didi Chuxing, and similar services. Conventional taxicabs relied on crude signaling mechanisms—literally, in this case, a would-be passenger attempting to wave down an empty cab in the street or calling a company’s dispatcher. These mechanisms created significant unmet demand. On the supply side, many cabs spent a large share of their time empty and cruising for passengers. Furthermore, most vehicles are underutilized; globally, most personally owned cars are in use for approximately 5 to 10 percent of waking hours.61 Excess supply sometimes pooled in certain spots, while other areas went largely underserved. For several reasons, including heavy regulation and static pricing, taxi markets were and continue to be highly inefficient. These inefficiencies—combined with the fact that the speed of hailing is of primary importance—made the market ripe for a radically different model to take root.
That model combined digital platforms with location-based mapping technology to instantly match would-be passengers with the driver in closest proximity. In addition, the location data can be analyzed at the aggregate level to monitor overall fluctuations in supply and demand. This allows for dynamic pricing adjustments, with price increases creating incentives for more drivers to work during periods of high demand. The platform nature of these services, which makes it easy for new drivers to join, unleashed flexible supply into the transportation market. Different types of mobility services have been launched, including not only ride sharing (such as Uber and Lyft) but also car sharing (Zipcar) and ride pooling (Lyft Line, UberPool).
From the outset, these platforms collected data from their user base to implement improvements—and as the user base grew, they generated even more data that the operators used to improve their predictive algorithms to offer better service. This feedback mechanism supported exponential growth. Uber, founded in 2009, is now in more than 500 cities and delivered its two billionth ride in the summer of 2016.62 Lyft reportedly hit almost 14 million monthly rides in July 2016.63 In China, ride-sharing giant Didi Chuxing now matches more than ten million rides daily. Today mobility services account for only about 4 percent of total miles traveled by passenger vehicles globally. Based on their growth momentum, this share could rise to more than 15 to 20 percent by 2030. This includes only real-time matching platforms and excludes the potential effects of autonomous vehicles.
The changes taking place in urban transportation—including a substantial hit to the taxi industry—may be only the first stage of an even bigger wave of disruption caused by mobility services. These services are beginning to change the calculus of car ownership, particularly for urban residents. Exhibit 7 indicates that almost one-third of new car buyers living in urban areas in the United States (the segment who travel less than 3,500 miles per year) would come out ahead in their annual transportation costs by forgoing their purchase and relying instead on ride-sharing services. For them, the cost of purchasing, maintaining, and fueling a vehicle is greater than the cost of spending on ride-sharing services as needed.
If we compare car ownership to car sharing instead of ride sharing, around 70 percent of potential car buyers could benefit from forgoing their purchase. A future breakthrough that incorporates autonomous vehicles into these services, thereby reducing their operating costs, could increase this share to 90 percent of potential car buyers in urban settings.
These trends are beginning to reshape the structure of the overall transportation industry. Value is already shifting from physical assets to data, analytics, and platforms as well as high-margin services such as matching. This is even playing out within the car-sharing market itself, as Car2Go, Zipcar, and other firms that own fleets now face newer platform- based players such as Getaround. Hyperscale platforms will likely create concentrated markets, since network effects are crucial to their success.
Economic impact and disruption
Hyperscale, real-time matching in transportation has the potential to generate tremendous economic impact. Individual consumers stand to reap savings on car purchases, fuel, and insurance by shifting to mobility services; they could also gain from having to spend less time looking for parking. Furthermore, the public will benefit from reduced real estate dedicated to parking, improved road safety, and reduced pollution. Summing these effects and assuming a 10 to 30 percent adoption rate of mobility services among low- mileage travelers, we estimate global economic impact in the range of $845 billion to some $2.5 trillion annually by 2025 (Exhibit 8). However, these shifts will create winners and losers. Some of the benefits will surely go to consumer surplus, while some will go to the providers of these platforms and mobility services.
The largest part of this impact stems from consumers shifting away from car ownership. By moving to mobility services, the average global consumer is likely to gain around $2,000 annually in cost savings over purchasing and maintaining a vehicle. With around 1.6 billion active vehicles projected by 2025, even 10 to 30 percent adoption of mobility services among low-mileage vehicle users can lead to $330 billion to $1 trillion in savings annually (this includes about $140 billion to $430 billion potentially realized in developed regions and $190 billion to $570 billion in developing regions).
In addition to direct car ownership costs, the shift toward mobility services will generate significant savings in related costs like parking. Consumers are projected to spend $3.3 trillion on parking services in 2025, but the use of mobility services could allow them to save $330 billion to $990 billion. Around $220 billion to $650 billion of this could be realized in developed countries and $110 billion to $340 billion in developing countries.
There is an additional benefit from the reduced demand for driving and parking. If 15 to 30 percent of drivers on the road in cities are looking for parking, this is a major logistical challenge in dense urban cores. By boosting the utilization of each vehicle, mobility services can decrease demand for parking and help reduce congestion, which creates further positive ripple effects on mobility and time saved. The reduced search for parking can generate a time-saving effect due to reduced congestion that can be valued at $10 billion to $20 billion as well as fuel savings in the range of an additional $20 billion to $60 billion.
Meanwhile, the shift to mobility services can improve productivity. Each day, workers spend 50 minutes in driving commutes on average in both developed and developing countries. If even half of that time can be used more productively for work, mobility services could generate an additional $100 billion to $290 billion in potential benefit.
Finally, ride sharing can improve road safety by creating a more viable option that keeps people from getting behind the wheel when they have been drinking, they are excessively tired, or they have other impairments (such as difficulties with night vision). Traffic accidents result in about 1.25 million deaths globally per year, with millions more sustaining serious injuries. One study found that ride-sharing services have reduced accidents by an average of 6 percent. Another found a 4 to 6 percent reduction specifically in drunk driving fatalities. We estimate that reduced accident rates due to the expansion of digital mobility services could save $50 billion to $160 billion in economic terms—not to mention the incalculable value of reducing the human toll of accidents.
Beyond their effect on traditional taxi services, mobility services could have wider impact. Automakers are the biggest question mark as the calculus of car ownership changes, particularly for urban residents. While sales will likely continue to grow in absolute numbers, the shift toward mobility services could potentially halve the growth rate of global vehicle sales by 2030 (Exhibit 9). In response, car manufacturers will likely need to diversify and lessen their reliance on traditional car sales. Many appear to be preparing for this future; partnerships are forming between traditional automakers and mobility service providers or other high-tech firms. Toyota, for example, recently invested an undisclosed amount in Uber. Several other cross-sector alliances have formed in recent years, such as Volkswagen with Gett, GM with Lyft, and Fiat with Google.
Autonomous vehicles, which appear to be on the horizon, could accelerate this wave of change. When self-driving cars are added into the equation, supply and demand matching could improve even further since these vehicles can have higher utilization rates. Car pooling may increase, and the cost of urban transportation could plummet. On the flip side, the demand for car purchases could fall further, and many people who make a living as drivers (nearly two million in the United States alone with the majority being truck drivers) could be displaced.
The role of data and analytics in transportation is not limited to urban centers. It can also improve the efficiency of trucking routes and handoffs in the logistics industry. Rivigo has applied mapping technology and algorithms to improve logistics efficiency in parts of India.
Hyperscale matching platforms could be transformative in other areas as well
While urban transportation has been profoundly altered by mobility services, it is only one area where digital platforms have had a major effect. Retail, social networking, music, and even dating have been revolutionized by the introduction of hyperscale platforms. And because other markets have the same kind of inefficiencies that characterized the traditional taxi market before the advent of these services, they, too, could be transformed if platforms reach critical mass. In energy markets, for instance, demand can fluctuate dramatically and frequently by time and by region. The current energy grid is ill-equipped to smooth out the spikes in peak demand with excess off-peak supply. But wider deployment of smart grid technology can address this inefficiency by using new sensor data to generate more dynamic matching of supply and demand, in part by allowing small, private energy producers (even individual homeowners) to sell excess capacity back to the grid. This technology is developing quickly: the United States alone has committed more than $9 billion in public and private funds toward smart grid technology since 2010. In the Netherlands, some startups are using the peer-to-peer model to match individual households directly with small providers (such as farmers) who produce excess energy. Vandebron, for instance, charges a fixed subscription fee to connect consumers with renewable energy providers; in 2016, this service provided electricity to about 80,000 Dutch households.
The markets for certain types of short-term labor services are also being redefined. Driving passengers is only one of the many types of services now being offered through digital marketplaces. Others include household chores and errands, data entry, and simple coding projects. Conventional platforms (even digital job boards such as Craigslist) allow for static requests. But now platforms can match available workers with requests for their services on demand. TaskRabbit, for example, serves 18 US cities, matching more than 70 percent of task requests with a local provider within five minutes. There are more than 30,000 “taskers” globally, and the average worker who participates takes on two or three jobs a day, five days a week. Recent research from MGI has found that already some 15 percent of independent workers in the United States and Europe have used digital platforms to earn income. The non-profit Samasource is seeking to bridge this market gap by breaking down larger digital projects into smaller discrete tasks that can be handled by remote workers in developing countries. As of 2016, almost 8,000 workers participated on this platform, increasing their earnings by more than three-and-a-half times.
Platforms such as TaskRabbit and Samasource quickly match underutilized supply (people looking for work) with demand. This can have productivity benefits for businesses, while creating a new avenue for individuals who need work to generate income. Previous MGI research found that some 850 million people across seven major economies alone are unemployed, inactive, or working only part time. Previously they had few options for rejoining the workforce or increasing their hours, but these types of platforms increase the range of flexible options available to them.
Source : The Age of Analytics: competing in a data-driven word. McKinsey Global Institute - December 2016
A lire également nos précédents billets :
- Plateformes, territoires et services publics
- Lorsque la somme des voitures individuelles devient une infrastructure de transport public
- La nouvelle économie du stationnement
Commentaires